Interested in leveraging Ginkgo Enzyme Services for your R&D? Get in touch here!
Enzyme Engineering and Artificial Intelligence: A New Frontier
Enzymes are the heroes of biotechnology, serving as biological catalysts that make life’s complex reactions look easy. Inside of the cell, enzymes direct the flow of molecules through metabolic pathways, orchestrating biological functions. Outside of their cellular context, enzymes have been co-opted for specialized roles in manufacturing, speeding up processes that would otherwise be painstakingly slow. In pharmaceuticals, enzymes are custom-engineered to act as targeted therapeutics. Whether in life sciences or industrial applications, enzymes elevate our ability to engineer processes and enact chemistries by facilitating reactions with speed and specificity.
For years, scientists have used a variety of tools to design and optimize these crucial biological components. Traditional methods have often hinged on exploiting evolutionary pressures—letting nature do the heavy lifting over generations and then picking the winners. Structure-based prediction techniques, like Rosetta, also made a significant impact, allowing researchers to model how tweaks to an enzyme’s structure could influence its activity.
But we’re entering a new era–one in which we can train Artificial Intelligence (AI) models based on large biological data sets. This is where Ginkgo Bioworks comes in. Our expansive cell engineering platform is a data-generating powerhouse, churning out the kind of high-quality, voluminous data that AI algorithms thrive on. The marriage of this large-scale data generation with AI models allows us to transcend previous limitations, making Ginkgo an ideal environment to train and deploy machine learning tools for the complex art of enzyme engineering.
The AI Story: Big Data, Bigger Breakthroughs
AI learns from large data sets. Ginkgo Bioworks generates these types of data: we make it possible for you to produce and learn from large data sets. Our extensive repositories of enzymes not only cover a wide range of protein sequences but are also complemented by highly targeted data, revealing precise sequence-function correlations. This dual-data approach is implemented through machine learning cycles in our enzyme engineering projects, enabling us to iteratively refine predictive models.
Ginkgo has developed an AI tool, Owl, to fine-tune enzymes for a specialized role. An expansive data set provides the foundational architecture. To construct the intricate details, however, we employ data that is calibrated to the specific enzyme and its intended function. This enables Owl, our machine learning tool, to not merely “learn” but to “apply” its learnings, writing the intricate, detailed novel enzyme that our scientists require. Owl can “see in the dark” and discern viable paths in complex enzyme design landscapes.
Ginkgo’s approach to enzyme design isn’t merely data accumulation; it’s strategic data deployment. Our Foundry is equipped to generate an extensive range of high-quality biological data at scale. From DNA design and synthesis to high-throughput screening, we create vast data sets corroborating structure-function relationships. Owl thrives in this environment, allowing us to design enzyme variants tailored to our partners’ unique specifications, whether that’s enzyme activity, specificity, or other parameters.
As we navigate the complexities of enzyme design and optimization, think of Owl as the expert navigator and our robust data sets and data-generating capabilities as the compass and map. Together, they form a symbiotic alliance that not only challenges but also redefines the boundaries of traditional R&D.
Tackling Enzymes in Central Carbon Metabolism: the power of iteration and integration
Enzymes that regulate flux through Central Carbon Metabolism (CCM) are biological masterpieces. These proteins have been shaped by billions of years of evolutionary refinement to execute their functions with unmatched precision and, in many cases, maintain high sequence and structure conservation throughout the tree of life.
In one example of Owl-guided enzyme optimization, we were asked to improve the reaction kinetics of an enzyme involved in CCM. While this enzyme had been studied for the past 50 years, the best improvement we found in the literature was a 2-fold increase in the kcat/KM–catalytic efficiency; our customer needed a 10-fold improvement in the efficiency of this enzyme in order to meet their economic targets.
Our approach to this project leveraged our Foundry’s ability to generate and test large libraries of strains. In our initial data-generation phase, we created a first-generation library featuring 2,000 distinct enzyme variants crafted using a structure-based design, as well as semi-rational methods like active-site mutagenesis for targeted alterations. This is an important step because it generated a data set for initial Owl training. With this information in hand, we designed a second generation library to give Owl more information: we maintained the library size of the first but incorporated insights from the previous round, resulting in an exciting 3.9-fold improvement—a leap that surpassed anything we had seen before.
But the real improvements were just beginning. The third generation of this program brought us to a pivotal point in our optimization journey. Leveraging Owl’s predictive analytics, we strategically developed a broad library of 4,000 enzyme variants, generating diversity where it mattered most. The result was an unprecedented 4.5-fold improvement in enzyme efficiency, serving as a testament to Owl’s growing mastery in predictive capability.
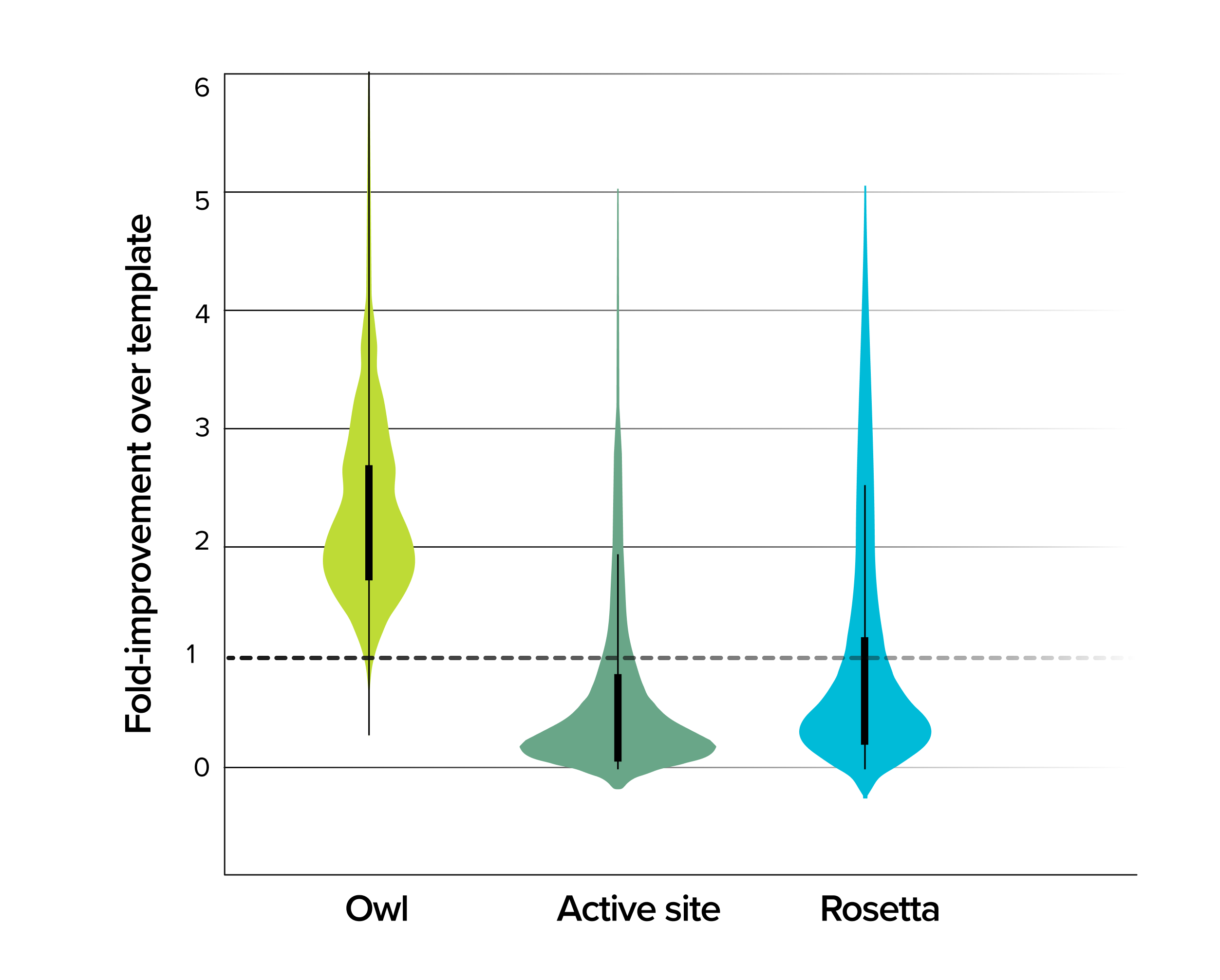
Data from these three consecutive generations positioned us to make our biggest improvements yet. Given the data that our scientists had generated, Owl continued to generate increasingly sophisticated models of enzyme function. The final iteration culminated in a fourth generation where only 100 enzyme variants needed to be tested. The result, which marked the successful completion of this customer program, was astonishing: a 10-fold improvement in enzyme function, verified through meticulous arrayed activity assays and detailed protein characterization. By integrating the large data sets generated by Ginkgo’s cell engineering platform with Owl’s predictive power, we surpassed the bounds of natural evolution and decades of research reported in the literature meet our customer’s targets.

The future of enzyme engineering: large data and machine learning at Ginkgo Bioworks
The confluence of big data and AI accelerates the pace of innovation to unprecedented speeds. Ginkgo’s cell engineering platform is an ecosystem designed for generating expansive, high-quality data sets customized for complex biological inquiries. This data, in turn, fuels the predictive power of AI models. Together, they form a symbiotic relationship that enables us to challenge the limitations of natural evolution and traditional research methods.
As stakeholders in the biotechnology industry, navigating complex R&D challenges requires more than just robust tools; it requires effective partnerships. Ginkgo Bioworks offers the specialized machine learning models and data-generation capabilities necessary to advance your research and overcome bottlenecks. Our suite of resources is designed to integrate seamlessly with your objectives, providing actionable insights and solutions tailored to your specific challenges.
Ginkgo is investing in the future of AI for biotech: see our recent announcement with Google about developing foundation generative AI models for DNA and protein. Leverage our expertise and technology for your next project, and to join us in pushing the boundaries of what is possible in synthetic biology.