Introduction
In a previous post Rapid Data Retrieval from Very Large Files, Eric Danielson discussed how Ginkgo’s software engineers are enabling fast access to massive sequence datasets. As a bioinformatician, I need file formats such as fasta
and gff
, for command line tools and biological sequence-processing libraries. However, these flat-file, semi-structured formats don’t scale to hundreds of millions of sequences. File compression makes data storage tractable, but finding and extracting a specific sequence among millions in a random position in the file has been a bottleneck.
Please read Eric’s full article, but the gist is that the block gzip (BGZip) from the samtools/htslib
project is the highest performing tool for getting (compressed) data out of a standard file store. BGZip’s “trick” is to concatenate multiple gzipped files together – where a single gzip block is no more than 64KB. A secondary index file, under GZI extension, is used to keep track of the locations of the blocks, with respect to the compressed and uncompressed bytes. BGZipped data is itself a valid gzip archive and thus, can be (de)compressed with any gzip library.
Using these tools, we can retrieve a sequence somewhere in the middle of a huge, bgzipped fasta
file (and we already know position of the first uncompressed byte), skipping directly to the relevant block – even if it’s at the end of the file – and only decompress the chunks that we actually need.
In practice, we usually won’t know ahead of time where a particular sequence is located in the file, but rather just its name. The fasta
format specifies that names and other meta-data are stored in the headers of the fasta
file, which are lines demarcated with a “>” character and thus marks the start of a new record. The actual sequence follows on the next line(s). Eric’s post also discussed creating a 3-column, tab-separated location file that is especially useful for fasta
files. This tracks the sequence name (i.e. pulled from the fasta
header), the uncompressed start position and the size (in bytes) of the full record. We’ll use a .loc
extension for this file.
We’ve used this file format in Ginkgo’s metagenomic data workflows together with htslib
’s bgzip
command line tool, which has options for specifying the position and number of characters of the desired place in the file.
So why did we want to improve on this already-excellent solution? Well, the bgzip
command line tool only has the option to extract one contiguous part of the file (one start position and its offset). We want to be able to retrieve potentially thousands of sequences from our fasta
files in one shot.
We had been handling this in the simplest way possible; a bash
script that loops through all the sequences/positions and calls bgzip. But it turns out that this is pretty inefficient (I’ll get to the specific timing results in a bit). Similarly, we’ve been building the location file by un-gzipping the library fasta
file and using a simple python loop to record the sequence name and position (and this doesn’t exploit the block GZip structure at all).
Ideally, we would implement our BGZip reading tools using the native C library from htslib
. But this has drawbacks – mostly in maintenance costs since we don’t have any C developers in our group. Kudos to samtools for open-sourcing this codebase, but it doesn’t meet our needs.
This is where Rust and the excellent noodles
crate, a collection of bioinformatics I/O libraries, comes in.
In the last few years, the Rust programming language has been taking the bioinformatics community by storm. It offers thread-safe, low level access to all your bits and bytes. Therefore, it’s the perfect use case for trying to squeeze out additional computational efficiency here, since we want fast reads from bgzipped files with as small a memory footprint as possible.
Using Rust and noodles
, we can create small, safe command line utilities for accessing our compressed data. And while Rust has a steeper learning curve than scripting languages like python, it’s not nearly as daunting as C in this use-case. Moreover, noodles
provides paralleling and asynchronous processing plugins for BGZip formats which will give us, for example, simultaneous parsing of independent data blocks.
Reading Sequences in BGZF-compressed fasta Files
Firstly let’s set up some test data which we’ll use going forward to obtain performance metrics. We’ll be using amino acid sequences from the same soil metagenomic library that Eric talked about in his last post. It contains 150 million sequences and the fasta
file is 235 gigabytes when uncompressed.
However, for this post, we don’t need such a large file just for benchmarking. These methods are all linear in computational complexity, so I’ll just grab a subset of the total:
$ grep -c ">" ca29_soil2_aa_sub.fasta
1166861
Let’s use bgzip
to compress (the -c
flag) and index (the -r
flag) the fasta
file:
$ bgzip -c ca29_soil2_aa_sub.fasta > ca29_soil2_aa_sub.fasta.gz
$ bgzip -r ca29_soil2_aa_sub.fasta.gz
The last command creates a binary index file called ca29_soil2_aa_sub.fasta.gz.gzi
in our working directory.
In our standard setup, we needed to decompress the gzip file (since we do not store uncompressed files) to get the locations of the fasta headers and length of the sequences (tracking the raw number of characters, including the header line). We can do that with a relative simple python script that keeps track of the current byte position of each new header and writes out a tab-separated locations file:
Let’s run that now and also keep track of the execution time.
$ time (
zcat < ca29_soil2_aa_sub.fasta.gz > ca29_soil2_aa_sub.fasta &&\
python fasta_indexer.py ca29_soil2_aa_sub.fasta
)
real 1m10.992s
user 0m31.566s
sys 0m3.770s
time
is a system command available in UNIX systems that tells us how long the program takes to run. The output we care about is “real” time, which is what the human user is experiencing.
The script fasta_indexer.py
made a location file called ca29_soil2_aa_sub.fasta.loc
. Let’s take a peek at it. Column 1 is the header names, Column 2 is the position of that sequence in the file and Column 3 is the size of the sequence:
$ head -n 2 ca29_soil2_aa_sub.fasta.loc && echo "..." && tail -n 2 ca29_soil2_aa_sub.fasta.loc
ca29_soil2_c51cf9d4-216c-4963-8f9b-65ee6b197a07_1 0 116
ca29_soil2_c51cf9d4-216c-4963-8f9b-65ee6b197a07_4 116 448
...
ca29_soil2_99a091d5-f352-4e66-8a50-3c48d2070870_19 324714018 187
ca29_soil2_e966a3b0-d674-47bb-b8b4-6db071c3e3f4_5 324714205 456
For timing, lets just say our sequences are randomly located in the file and so we can just subsample ca29_soil2_aa_sub.fasta.loc for the simulation. 3000 sequences should be the right size for this demo:
shuf -n 3000 ca29_soil2_aa_sub.fasta.loc | sort -k2n > query_loc.txt
Now let’s run the bgzip
extraction code, accessing each sequence via the -b
/-s
(offset/size) command line options from within a bash loop:
$ time (
while IFS= read -r loc_id
do
## converts loc file to bgzip arguments
offset=$( echo "$loc_id" | cut -f2 )
size=$( echo "$loc_id" | cut -f3 )
bgzip -b $offset -s $size ca29_soil2_aa_sub.fasta.gz >> ca29_query.fasta
done < query_loc.txt
)
real 0m31.408s
user 0m10.102s
sys 0m14.933s
Can we do better than a bash loop by rewriting our BGZip reader to process all of our desired sequence locations in one go?
Let’s give it a try: It turns out that the IndexedReader
from the noodles-bgzf
sub-crate. Here we simply need to adapt the example code for seeking an uncompressed position into a loop that does the same thing. We’ll call this program bgzread
:
// bgzread
use std::{
env,
io::{self, BufRead, BufReader, Read, Seek, SeekFrom, Write},
fs::File
};
use noodles_bgzf as bgzf;
fn main() -> Result<()> {
// Gather positional cli argument for the input files
let mut args = env::args().skip(1);
let src = args.next().expect("missing src");
let loc = args.next().expect("missing location");
// Set up the readers for the compressed fasta and location files
let mut reader = bgzf::indexed_reader::Builder::default().build_from_path(src)?;
let loc_reader = File::open(loc).map(BufReader::new)?;
// We'll be writing the final data to standard out
let mut writer = io::stdout().lock();
// Loop through the location reader to get positions
for line in loc_reader.lines() {
// parse the lines
let line = line.unwrap();
let (raw_position, raw_length) = line.split_once(" ").unwrap();
let pos: u64 = raw_position.parse()?;
let len: usize = raw_length.parse()?;
// Seek start position and read 'len' bytes into the buffer
reader.seek(SeekFrom::Start(pos))?;
let mut buf = vec![0; len];
reader.read_exact(&mut buf)?;
// Write the outputs
writer.write_all(&buf)?;
}
Ok(())
}
Dare I claim that this is a pretty darn simple program? We have to do a little bit of explicit typing for the position
and len
variables and all the ?
and unwrap
statements are a bit mysterious. But basically, this is how Rust language gets developers to be explicit about what types of data we’re passing around and to handle potential errors. In this example, we’ll just throw the built-in panic
message if, say the user-specified input file doesn’t exist.
Full disclosure that this isn’t the exact bgzread
script we have in production at Ginkgo – we’ve got some customized error handling and other file buffering tricks. But this will be good enough for the demo.
The other nice part about Rust, is that the cargo
packaging system compiles our program, taking care of linking libraries and dependencies. We can just ship the standalone binary to our users.
Now that I’ve covered the basics, we can get to the good stuff:
$ time bgzread ca29_soil2_aa_sub.fasta.gz query_loc.txt > ca29_query.fasta
real 0m4.057s
user 0m1.165s
sys 0m0.158s
Based on the above time results we can get ~5x speedup in our demo sequences by re-implementing the loop in Rust!
We’ll get to why this is so much faster a bit later. For now I’ll just say that it’s really nice to have control over all the BGZF classes, which are natively implemented by noodles-bgzf
.
You’ll notice that we’re also using BufReader so that we can dump decompressed, buffered outputs to stdout so that we never have to hold much data in memory.
I’ll also mention that this bgzip reader can also be made multi-threaded (which I’m not showing in the example code). Since the gzip blocks are independent, we can potentially access and decompress multiple blocks simultaneously. I won’t run that here, since the datasets are small enough that the parallelization overhead isn’t worth it. Later, we will see an example of thread-safe file access that we get in Rust.
What is the performance of bgzread
in the wild? We have a Nextflow pipeline called nextflow-fasta-selector
to look up sequences from their names (you don’t need to know the locations ahead of time). In our test case, we wanted to look up 20k sequences from the full ca29 contig library:

Astonishingly, this results in a 14.8x speedup, and we are reading 240x less data!
The bgzip+bash loop is not quite so bad as it looks here since in production, we can actually split up the 20k sequences into chunks of around 500-1k sequences and batch-process them. However, it is clearly preferable not to have to set up a single bgzip CLI call for every seek + read/deflate operation we need to do.
Understanding GZI Files
Under the hood, BGZF uses another file to track the position of the independently-compressed GZip blocks, called the gzip-index or GZI file. This file uses the .gzi
extension. It consists of the compressed and uncompressed offsets of the blocks, as 64-bit unsigned integers – and starting with the second block of course, the first block will always start at (0, 0). But don’t bother trying to read this file directly, fellow human, it’s in a binary format.
Earlier in this post, I made the claim that part of what we gain by using noodles is not just that Rust loops are faster than BASH loops, but that by writing our own program, we only have to read the GZI file once. Our script gets the GZip block positions, and then we can look up our sequences iteratively within those blocks. In bgzip, we have to read in the GZI file independently every time we want to read a position.
Let’s take a look at how long each program is spending reading this file. We can do this using strace, a tool available in Linux. Since both bgzip’s C routines and Rust’s noodles library are at the end of the day using the system calls to read files, strace can tell us when those calls are happening.
For strace, we’ll alias a command that asks traces of openat
, reads and close file operations and the flags -ttt
will give us timestamps. Finally, we’ll pipe the log (which would normally go into stdout
) into a file:
$ alias stracet="strace -ttt -o >(cat >strace.log) -e trace=openat,close,read"
Now let’s call this command for a single bgzip run, for an arbitrary sequence and parse the log file to see where the gzi
is getting open and read:
$ stracet bgzip -b 112093709 -s 1149 ca29_soil2_aa_sub.fasta.gz > seq.fa
$ sed -rn '/openat\(.*\.gzi\"/,/close/p' strace.log
1677366815.387446 openat(AT_FDCWD, "ca29_soil2_aa_sub.fasta.gz.gzi", O_RDONLY) = 5
1677366815.388736 read(5, "n\23\0\0\0\0\0\0$\221\0\0\0\0\0\0\0\377\0\0\0\0\0\0^\"\1\0\0\0\0\0"..., 4096) = 4096
1677366815.389304 read(5, "\0\0\377\0\0\0\0\0\311\2\220\0\0\0\0\0\0\377\377\0\0\0\0\0\7\221\220\0\0\0\0\0"..., 4096) = 4096
1677366815.389667 read(5, "\0\0\376\1\0\0\0\0h\215\37\1\0\0\0\0\0\377\376\1\0\0\0\0\370\34 \1\0\0\0\0"..., 4096) = 4096
1677366815.389938 read(5, "\0\0\375\2\0\0\0\0\201$\257\1\0\0\0\0\0\377\375\2\0\0\0\0\233\266\257\1\0\0\0\0"..., 4096) = 4096
1677366815.390206 read(5, "\0\0\374\3\0\0\0\0c\233>\2\0\0\0\0\0\377\374\3\0\0\0\0\27*?\2\0\0\0\0"..., 4096) = 4096
1677366815.390468 read(5, "\0\0\373\4\0\0\0\0/\331\315\2\0\0\0\0\0\377\373\4\0\0\0\0>g\316\2\0\0\0\0"..., 4096) = 4096
1677366815.390690 read(5, "\0\0\372\5\0\0\0\0hZ]\3\0\0\0\0\0\377\372\5\0\0\0\0Z\351]\3\0\0\0\0"..., 4096) = 4096
1677366815.391017 read(5, "\0\0\371\6\0\0\0\0y\376\354\3\0\0\0\0\0\377\371\6\0\0\0\0U\216\355\3\0\0\0\0"..., 4096) = 4096
1677366815.391344 read(5, "\0\0\370\7\0\0\0\0\236\230|\4\0\0\0\0\0\377\370\7\0\0\0\0\270*}\4\0\0\0\0"..., 4096) = 4096
1677366815.391668 read(5, "\0\0\367\10\0\0\0\0\311\34\f\5\0\0\0\0\0\377\367\10\0\0\0\0\335\254\f\5\0\0\0\0"..., 4096) = 4096
1677366815.391938 read(5, "\0\0\366\t\0\0\0\0\206\217\233\5\0\0\0\0\0\377\366\t\0\0\0\0\347\35\234\5\0\0\0\0"..., 4096) = 4096
1677366815.392226 read(5, "\0\0\365\n\0\0\0\0\212\16+\6\0\0\0\0\0\377\365\n\0\0\0\0A\237+\6\0\0\0\0"..., 4096) = 4096
1677366815.392492 read(5, "\0\0\364\v\0\0\0\0r\246\272\6\0\0\0\0\0\377\364\v\0\0\0\0'6\273\6\0\0\0\0"..., 4096) = 4096
1677366815.392724 read(5, "\0\0\363\f\0\0\0\0\276\37J\7\0\0\0\0\0\377\363\f\0\0\0\0\217\257J\7\0\0\0\0"..., 4096) = 4096
1677366815.393001 read(5, "\0\0\362\r\0\0\0\0z\231\331\7\0\0\0\0\0\377\362\r\0\0\0\0\f,\332\7\0\0\0\0"..., 4096) = 4096
1677366815.393291 read(5, "\0\0\361\16\0\0\0\0\270\\i\10\0\0\0\0\0\377\361\16\0\0\0\0\355\354i\10\0\0\0\0"..., 4096) = 4096
1677366815.393542 read(5, "\0\0\360\17\0\0\0\0.\311\370\10\0\0\0\0\0\377\360\17\0\0\0\0\226V\371\10\0\0\0\0"..., 4096) = 4096
1677366815.393788 read(5, "\0\0\357\20\0\0\0\0\210g\210\t\0\0\0\0\0\377\357\20\0\0\0\0\330\370\210\t\0\0\0\0"..., 4096) = 4096
1677366815.394110 read(5, "\0\0\356\21\0\0\0\0\f\342\27\n\0\0\0\0\0\377\356\21\0\0\0\0\23t\30\n\0\0\0\0"..., 4096) = 4096
1677366815.394317 read(5, "\0\0\355\22\0\0\0\0~u\247\n\0\0\0\0\0\377\355\22\0\0\0\0d\4\250\n\0\0\0\0"..., 4096) = 1768
1677366815.394678 close(5) = 0
We can see the gzi
file being open, and the bytes being read into the bgzip program’s buffer and finally closed. Each operation is preceded by a UTC timestamp that we can use to calculate the how much time (in seconds) the program is operating on the file:
$ gzitime () {
timestamps=( `sed -rn '/openat\(.*\.gzi\"/,/close/p' $1 |
awk -v ORS=" " 'NR == 1 { print $1; } END { print $1; }'` )
bc <<< "${timestamps[1]} - ${timestamps[0]}"
}
A short explanation of the above bash
function: we’re opening the log file and finding all the lines between opening and closing the gzi
file, as before. Then, we’re using awk
to grab the first and last timestamps to calculate the time between those operations (which is all the file reads) via the basic calculator (bc
) shell command.
$ gzitime strace.log
.007232
7.2 milliseconds doesn’t seem like a lot, but do that again and again, over 3000 independent runs, and it explains what our bash
-looped bgzip program is spending over half of its time doing.
Compare this to our Rust program:
$ stracet bgzread ca29_soil2_aa_sub.fasta.gz <( echo "112093709 1149" ) > seq.fa
$ gzitime strace.log
.004572
Not only does this read the index a bit faster than bgzip; it also only runs once!
Indexing the Locations of Sequences in Compressed fasta Files
Clearly, we have already got some significant and actionable performance gains by implementing our own tool in Rust. This is useful, but it turns out that generating the location file for the header positions in the block GZipped-formatted fasta
file is actually an even bigger bottleneck than reading locations.
Could we also turn that python script into a Rust command line tool?
I won’t show the whole program (so please don’t try to compile this at home!) but here is a snippet to give you a flavor:
// bgzindex snippet
while let Ok(num) = reader.read_line(&mut line) {
// Supressed code: updating block_i //
// Get current block offset from global index //
block_offset = bgzf_index[block_i];
if line.starts_with('>') {
if size > 0 {
// Write the size of the previously found record
write_size(&mut writer, size);
}
// We found a new record so reset size counter
size = 0;
// Write the current record name
let name: &str = &line.to_string();
write_name(&mut writer, &name[1..(num-1)]); //subset to trim out < & newline
// Write the current position
let virtual_pos = block_offset + (reader.virtual_position().uncompressed() as u64) - (num as u64);
write_pos(&mut writer, virtual_pos);
}
size += num;
line.clear(); // Clear the line buffer for the next line
}
In this program, we need to keep track of three pieces of data: the sequence name (from the fasta
header); the start position of the uncompressed sequence record (pos
– position); and size
(the number of uncompressed bytes following the start position). We have three convenience functions that I’m not showing the bodies of — write_name, write_pos and write_size — but these simply handle writing the output in the correct three column format.
To summarize the program, whenever we encounter a line that starts with a < character we know we’ve hit a new record. So, we’ll write out the size of the previous record, if one exists yet. Then, we’ll write out the new name – the whole header line except the start token and the final newline. The size
(the number of uncompressed characters) of the final sequence record is also easy – we just need to keep a running sum of the size of all the lines associated with that sequence record.
Now the tricky part: we need to calculate the start position. In the python version of this code, we uncompressed the entire file first, so we could just use one global, position tracker. However in the Rust implementation, we want to take advantage of the block format. So, we’ll use the BGZip index (stored in the gzi
file) to calculate a global offset relative to the virtual_position
of the reader within the block and the start of the block_offset
. We’ll just need to keep track of which block we’re in — that’s the block_i
indexing variable — and use a local copy of bgzf_index, which is just a vector containing the uncompressed block start positions, which is read directly from the gzi
file.
Finally, since reader.read_line
function puts our cursor at the end of the line, we need to subtract the line length, num
, to get the start position.
Let’s see how this program does:
$ time bgzindex ca29_soil2_aa_sub.fasta.gz ca29_soil2_aa_sub.fasta.loc
real 0m14.650s
user 0m2.745s
sys 0m1.328s
Which is a very nice speedup over the Python script (which took over a minute).
We can even try to parallelize the indexing over multiple threads:
$ time bgzindex ca29_soil2_aa_sub.fasta.gz ca29_soil2_aa_sub.fasta.loc 2
real 0m12.395s
user 0m2.976s
sys 0m0.966s
That’s a 6x speedup all told! Additional cores don’t seem to help much beyond two, however.
And of course the location file looks the same as before:
$ head -n 2 ca29_soil2_aa_sub.fasta.loc && echo "..." && tail -n 2 ca29_soil2_aa_sub.fasta.loc
ca29_soil2_c51cf9d4-216c-4963-8f9b-65ee6b197a07_1 0 116
ca29_soil2_c51cf9d4-216c-4963-8f9b-65ee6b197a07_4 116 448
...
ca29_soil2_99a091d5-f352-4e66-8a50-3c48d2070870_19 324714018 187
ca29_soil2_e966a3b0-d674-47bb-b8b4-6db071c3e3f4_5 324714205 456
Our new bgzindex
has some details that lets it take advantage of multithreading. Primarily, we are using the gzi
(block gzipped index) file to keep track of the reader’s current virtual position relative to the current block. This adds a bit of overhead at first (we need to read and store the data in the block indexes) but it means that we will always know our global position from within the block we are reading from.
This is important because:
- We can ditch the global position tracker needed in the Python version of the script, which frees us up to do multithreaded gzip block decompression.
- Therefore, we avoid annoying the Rust borrow checker by trying to do something like change the value of the reader after it has been “moved” (e.g. read into the buffer).
Let’s try this out on a real-word hard case, indexing the locations of the amino acid sequences in the entire ca29 library.
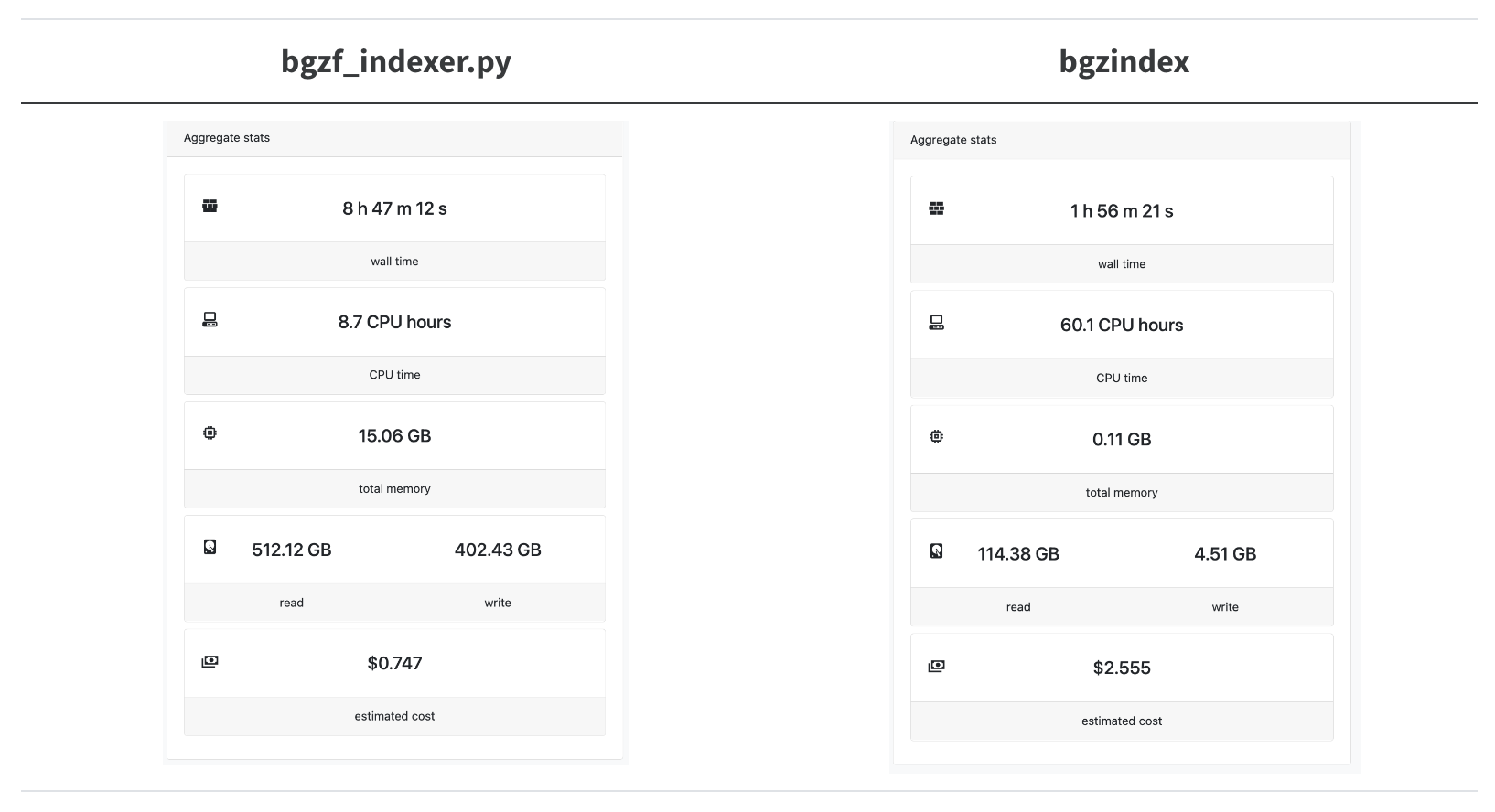
A 4.5x speedup! We’re trying to parallelize as much as possible so we overshot the mark for how many CPUs bgzindex
needs (and we overpaid for an instance in this case).
Concluding Remarks
Overall, I’ve been impressed at how easy it has been for me to get up running on Rust. Thanks to reliable, community-supported packages like noodles, I’ve been writing high-performing code after just a few months of learning the basics from “the book”.
I’ve been able to write code that is easy to read, simple to package up and distribute, and has allowed us to crunch through our massive datasets even faster and more reliably.
(Feature photo by Joshua Sortino on Unsplash)